
We use the population correlation function as a statistical measure for the hydrogen-bond lifetime. In an attempt to understand the complicated hydrogen-bonding, we developed new molecular visualization tools within the Vish Visualization shell and used it to visualize the life of each individual hydrogen-bond.
Esb Revisited 1080P Could Well
Liquid ethylene glycol at room temperature does not show significant clustering or chain building. The hydrogen-bonds break often due to the rotational and vibrational motions of the molecules leading to an H-bond half-life time of approximately 1.5 ps. However, most of the H-bonds are reformed again so that after 50 ps only 40% of these H-bonds are irreversibly broken due to diffusional motion. ESB:R 1080p could well be ready in a couple of months after i can start work properly on it. But now I come to the biggest problem with the upscale. Just rendering ESB:R as an upscale using uncompressed image files, and a few test renders of replacements, it almost filled a 4TB drive.
The work was preceded by a careful check of various OPLS-based force fields used in the literature. It was found that they lead to quite different angular and H-bond distributions.Enterprise Integration Patterns Revisited in 2014. Today, I had a talk about 'Enterprise Integration Patterns (EIP) Revisited in 2014' at Java Forum Stuttgart 2014, a great conference for developers and architects with 1600 attendees.
Esb Revisited Code Streams Of
What are the consequences of chaos for how such networks encode streams of temporal stimuli? On the one hand, chaos is a strong source of randomness, suggesting that small changes in stimuli will be obscured by intrinsically generated variability. Of A New Hope: Revisited (the coolest SW fan edit ever, see fanedit.org for details), decided he was going to make the TIEs all gray in ESB: Revisited.Highly connected recurrent neural networks often produce chaotic dynamics, meaning their precise activity is sensitive to small perturbations. There is an interesting post. Technology selection is notoriously difficult in the enterprise space since the criteria and complexity of the problem is often not fully understood until later in the development process.
A conclusion is that the presence of strong chaos in recurrent networks need not exclude precise encoding of temporal stimuli via spike patterns.Enterprise Integration Patterns Revisited (EIP, Apache Camel, Talend ESB) Technology, Education. Moreover, recurrence serves to distribute signals throughout chaotic networks so that small groups of cells can encode substantial information about signals arriving elsewhere. Here we show that strongly chaotic networks produce patterned spikes that reliably encode time-dependent stimuli: using a decoder sensitive to spike times on timescales of 10’s of ms, one can easily distinguish responses to very similar inputs.
In this article we revisit the implications of chaos in input-driven networks and directly measure its impact on evoked population spike patterns. As a result, the precise spiking patterns produced by such networks would be expected to be too fragile to carry any valuable information about stimuli, since inevitable sources of noise such as synaptic failure or ion channel fluctuations would be amplified by chaotic dynamics on repeated trials. Many theoretical studies found this firing regime to be associated with chaos, where tiny perturbations explode to impact subsequent neural activity. However, the realization of integration scenarios is a complex and time-consuming task.Baptista et al.: Archaeobuthus Revisited 29 Figure 43: Archaeobuthus estephani.Pedipalpal patella showing four external trichobothria, eb1, esb1, est, and et1.Recurrently connected populations of excitatory and inhibitory neurons found in cortex are known to produce rich and irregular spiking activity, with complex trial-to-trial variability in response to input stimuli.
This means synaptic currents nearly cancel on average, but feature strong fluctuations, giving rise to sustained irregular spiking. It is believed that recurrent cortical circuits typically operate in a “balanced state” in which every neuron receives a large number of excitatory (E) and inhibitory (I) inputs (see, e.g., ). We propose that chaos is a manageable by-product of recurrent connectivity, which serves to efficiently distribute information about stimuli throughout a network.Highly recurrent connectivity occurs throughout the brain. This can be explained by the presence of low-dimensional, input-specific chaotic attractors, leading to a form of trial-to-trial variability that is intermittent, rather than uniformly random.
How can stimuli be encoded in the variable spike trains that result (c.f. As a consequence, when the same stimulus is presented to a chaotic network multiple times, it may fail to generate reproducible responses. Chaos implies that the network dynamics depend very sensitively on network states, so that tiny perturbations to initial conditions may lead to large effects over time.
Arrow indicates a perturbation to the network on the second trial, in which an extra spike is “added” to neuron number 1. ( B) Raster plot of the network response to a fixed input I( t), on two trials. Can a network this sensitive to small differences in its internal state produce sufficiently reliable outputs for discrimination? If we were to present two different stimuli to such a network, could a decoder be trained to discriminate the spikes evoked by the first input from the spikes evoked by the second, despite both sets of output spikes being subject to the type of variability shown here? This is the central question that we investigate here.( A) An input I( t), with N independent components, is presented to a chaotic network of N spiking cells. This small perturbation quickly reverberates throughout the whole network in a seemingly random fashion. In each of these trials, the exact same stimulus is presented, but on the second trial a small perturbation is artificially introduced in the form of an extra spike for neuron 1 (as in ).
We relate this coding precision to previous work grounded in the mathematical theory of dynamical systems, which shows that—at the level of multi-neuron spike patterns—chaotic networks do not produce as much variability as one might guess at first glance. We find that, despite chaos, the network’s spike patterns encode temporal features of stimuli with sufficient precision so that the responses to close-by stimuli can be accurately discriminated. The strength of stimulus inputs is comparable to network interactions, so that dynamics are not dominated by external stimuli alone.
Indeed, chaos may represent a mechanism by which other sources of variability are amplified. Even though there are many likely contributors to this variability, ranging from stochasticity in neurotransmitter release or ion channels (, but see ) to confounding factors like behavioral state, activity level, and levels of adaptation , chaotic interactions may play an inescapable role. We also demonstrate that classical mean-field theories describe the overall firing statistics in our networks, but that additional tools are needed to describe their response to temporal stimuli.Our results are based on numerical simulations guided by mathematical theory, but connect to a broad experimental literature: trial-to-trial variability in neural responses to repeated stimuli is often observed empirically (though see also ). We quantify this via a chaos-induced “noise floor” stimuli whose strength exceeds this floor will be easily discriminable.We show that these findings are consistent with well-understood properties of irregular activity produced in balanced networks. Our main findings are:It is possible for strongly chaotic recurrent networks to produce multi-cell spike responses that remain discriminable even for highly similar temporal inputs.The same recurrent connections that produce chaos distribute stimulus information throughout the network, so that stimuli can be discriminated based on only a small subset of “readout” neurons.Input statistics influence the strength of chaotic fluctuations that can obscure stimuli.
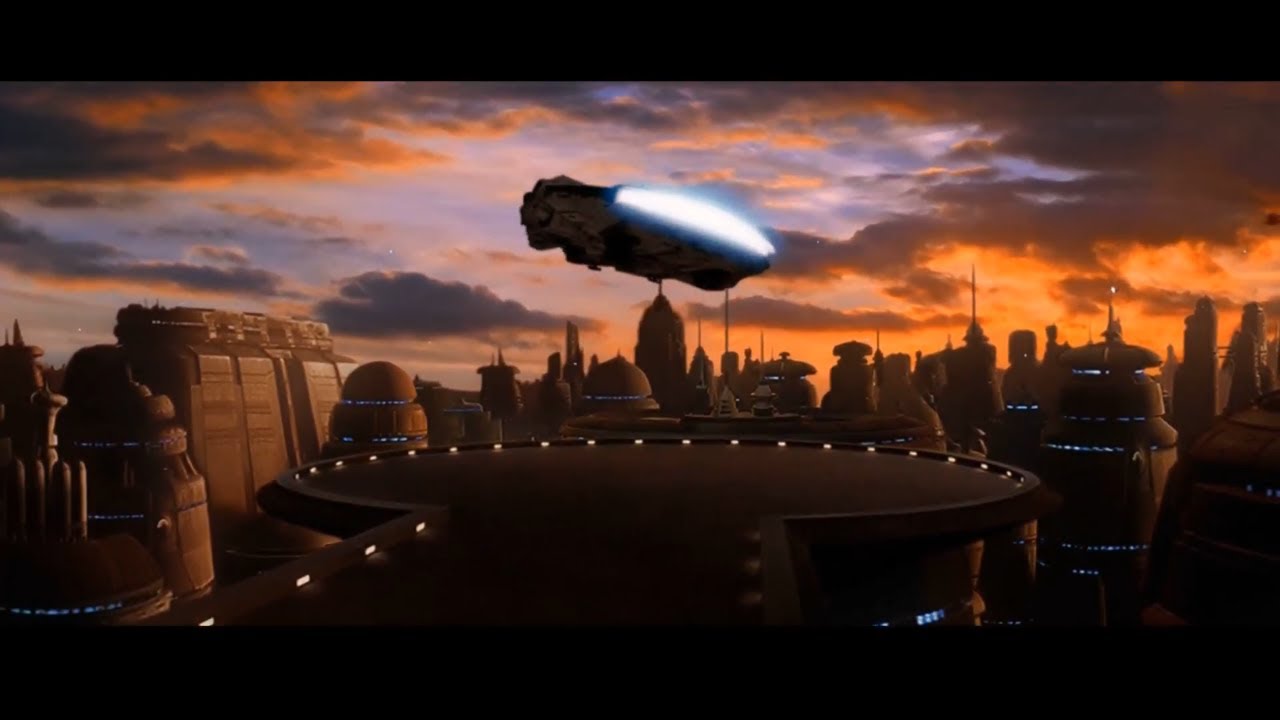
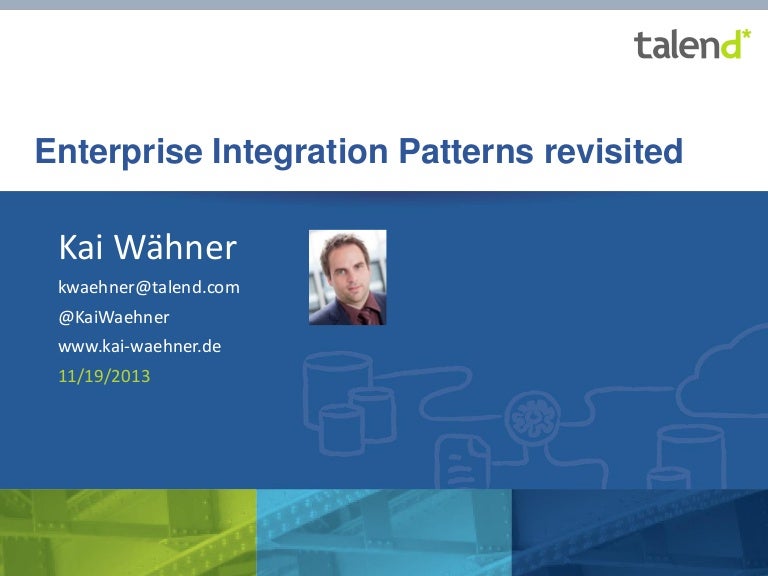
